Mastering Time Series Econometrics: 5 Powerful Models with Real-World Insights
Unlock the full potential of economic forecasting by learning time series econometrics — from ARIMA to GARCH, real-world examples, and advanced techniques.
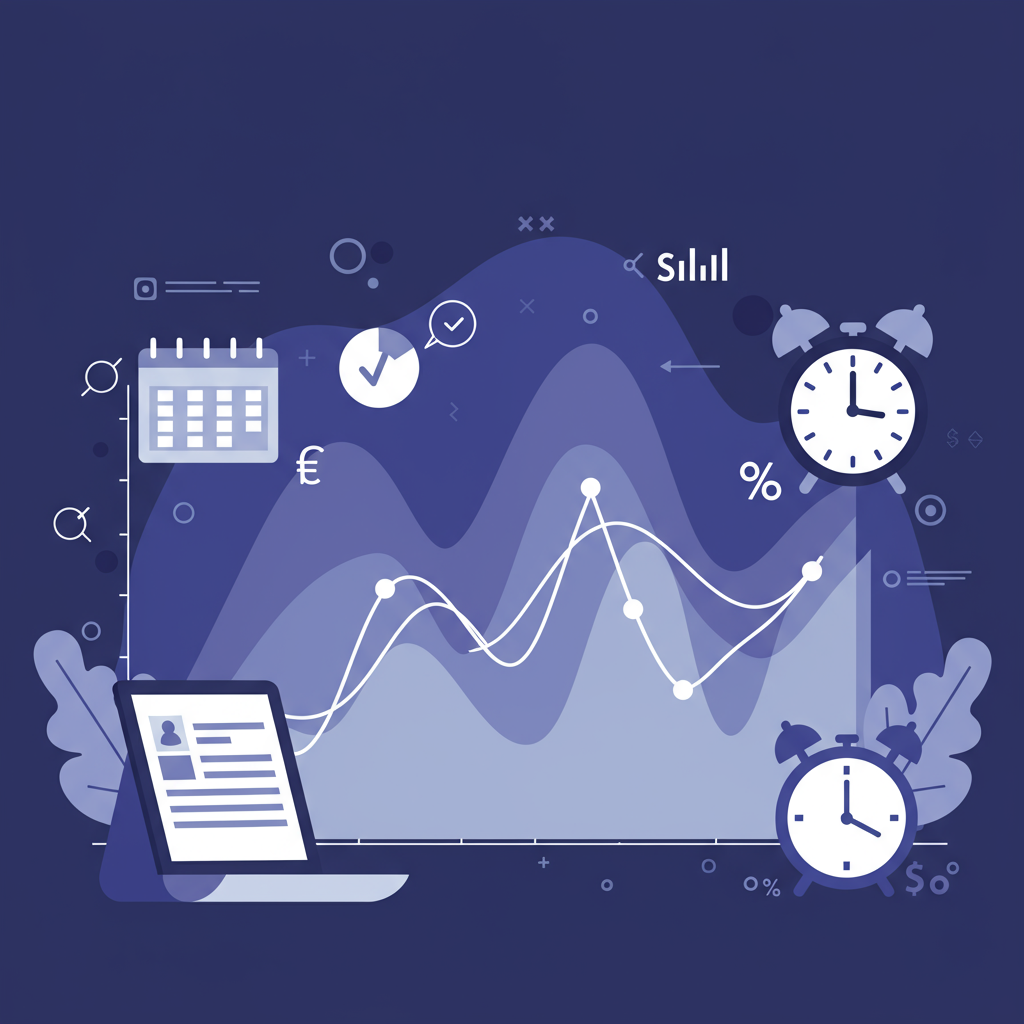
Contents
Table of Contents
- What is Time Series Econometrics?
- Core Concepts in Time Series Econometrics
- Top 5 Time Series Econometrics Models
- Practical Applications
- Common Challenges and Solutions
- Conclusion
What is Time Series Econometrics?
Time series econometrics is a field of quantitative economics that analyzes time-ordered data points to identify trends, cycles, and forecasting models. Unlike cross-sectional data, time series focuses on observations over intervals — daily, monthly, quarterly, or yearly — making it crucial for understanding economic dynamics.
Governments, businesses, and financial institutions use time series models to forecast inflation, predict market trends, and evaluate economic policies. With the growing availability of data and computing power, time series econometrics has become more relevant than ever in decision-making and forecasting.
Core Concepts in Time Series Econometrics
Before delving into models, it’s essential to understand key concepts that underlie most time series econometric analyses:
- Stationarity: A process is stationary if its statistical properties (mean, variance, autocorrelation) are constant over time. Non-stationary data must often be transformed using differencing.
- Autocorrelation: Refers to the correlation of a variable with its past values. It is a central element in autoregressive models.
- Lag Structure: Many economic relationships are not instantaneous; lagged variables help capture delayed effects.
- Seasonality: Repetitive patterns in time series (e.g., retail sales spikes in December) must be modeled explicitly.
- Shocks and Persistence: Measures how long an external shock (e.g., oil price spike) affects a time series.
Top 5 Time Series Econometrics Models
1. ARIMA (AutoRegressive Integrated Moving Average)
ARIMA combines autoregression (AR), differencing (I), and moving averages (MA) to model univariate time series. It’s highly effective for data with trends but no seasonality. For example, ARIMA is commonly used to predict unemployment rates and GDP growth.
2. SARIMA (Seasonal ARIMA)
Extends ARIMA by accounting for seasonality. SARIMA is widely applied in industries like retail or tourism where seasonal effects are dominant. The model includes seasonal autoregressive and moving average terms.
3. VAR (Vector Autoregression)
VAR allows modeling multiple interrelated time series. For instance, a central bank might model inflation, interest rate, and exchange rate simultaneously. It captures feedback loops between variables, unlike univariate models.
4. GARCH (Generalized Autoregressive Conditional Heteroskedasticity)
GARCH models volatility clustering, a phenomenon where large price changes are followed by more large changes. It’s especially useful in finance, where understanding volatility is critical for risk management and asset pricing.
5. State Space Models and Kalman Filter
These dynamic models are ideal for time-varying systems or when data is noisy. The Kalman Filter updates estimates recursively and is widely used in macroeconomic forecasting, especially for latent variables like potential output.
Practical Applications
- Macroeconomic Forecasting: Central banks use ARIMA and VAR to forecast inflation, employment, and output.
- Stock Market Volatility: Financial analysts apply GARCH models to predict market risk and inform portfolio decisions.
- Energy Demand: Power companies forecast electricity demand using SARIMA, considering daily and seasonal variations.
- Policy Impact Analysis: Time series econometrics helps evaluate the long-run effects of monetary and fiscal policies.
- Climate Change Economics: Models estimate CO₂ emission patterns and assess environmental policies.
Real-world decisions often depend on reliable forecasts. By applying appropriate time series models, economists can quantify uncertainty and design more effective policies.
Common Challenges and Solutions
- Non-stationary Data: Use unit root tests like Augmented Dickey-Fuller (ADF) to detect and transform non-stationary series.
- Model Overfitting: Avoid using too many parameters. Use AIC or BIC for model selection and validate with out-of-sample data.
- Multicollinearity in VAR: Standardize or reduce variables using techniques like PCA to avoid redundancy.
- Structural Breaks: Apply Chow test or switch to regime-switching models like Markov Switching VAR (MSVAR).
- Residual Diagnostics: Always perform diagnostic tests (e.g., autocorrelation, heteroskedasticity) post-estimation.
Addressing these challenges is crucial for developing robust and interpretable models that generalize well to unseen data.
Conclusion
Time series econometrics enables analysts to extract valuable insights from temporal data. By mastering key models like ARIMA, SARIMA, VAR, GARCH, and Kalman Filters, professionals can build predictive tools that support real-world economic decisions.
Whether you’re a policy analyst, investor, or academic, understanding the foundations and applications of time series econometrics will enhance your ability to anticipate trends, assess risk, and influence outcomes.