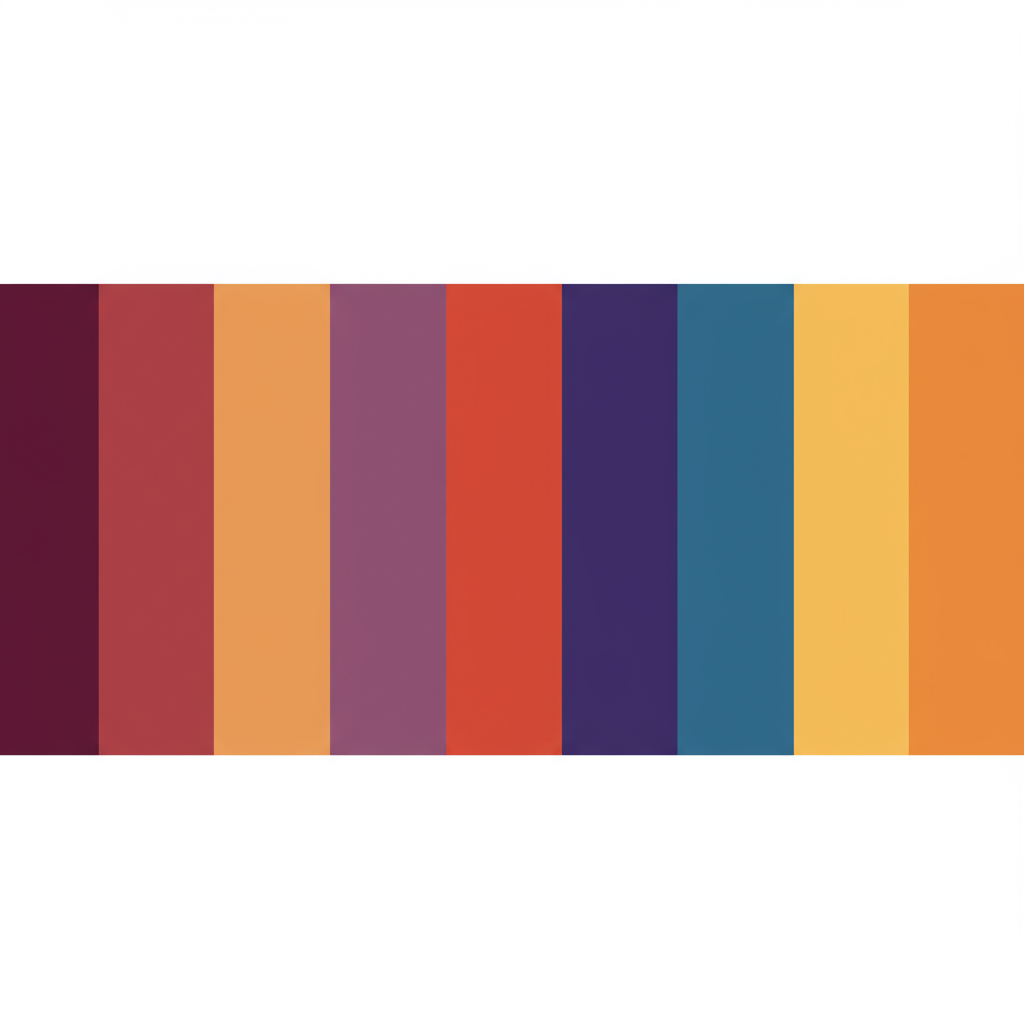
Understanding Cross-Sectional Econometric Analysis
A cross-sectional econometric analysis examines data collected from multiple subjects—such as individuals, firms, or countries—at a single point in time. Unlike time-series or panel data, it does not track changes over periods but provides a snapshot of relationships among variables.
This method is commonly used in labor economics, health economics, and consumer behavior studies where data is observed only once per subject.
Applications and Advantages
Economists and researchers apply cross-sectional econometric analysis to:
- Measure income inequality within a region
- Estimate consumer spending patterns
- Understand demographic influences on labor market participation
Advantages of this analysis include relatively simple data collection, fast results, and cost-effectiveness. Moreover, cross-sectional models are ideal for identifying correlations and testing economic hypotheses quickly.
Estimation Techniques Used in Cross-Sectional Econometrics
The most commonly used estimation technique in cross-sectional econometric analysis is the Ordinary Least Squares (OLS) method. OLS estimates the relationship between a dependent variable and one or more independent variables.
Key assumptions of OLS in cross-sectional settings include:
- Linearity in parameters
- No perfect multicollinearity
- Zero conditional mean of error terms
- Homoscedasticity
Model Specification and Diagnostic Checking
Proper model specification is critical. Omitting relevant variables or including unnecessary ones can lead to biased and inconsistent estimators. After estimation, diagnostic tests such as the Breusch-Pagan test for heteroskedasticity or the Ramsey RESET test for functional form should be conducted to validate results.
Real-World Example of Cross-Sectional Econometric Analysis
Let’s consider a cross-sectional dataset on household consumption and income. By running a regression where consumption is the dependent variable and income, education, and household size are independent variables, one can assess marginal propensities to consume across demographic groups.
Data can be obtained from sources like the Investopedia data repository or academic databases such as JSTOR.
Best Practices in Cross-Sectional Analysis
To enhance validity and reliability, researchers should:
- Use robust standard errors to correct for heteroskedasticity
- Perform sensitivity analysis by altering model specifications
- Cross-validate results using sub-sample analysis
- Interpret coefficients in the context of real-world implications
Common Challenges and How to Address Them
Conducting accurate cross-sectional econometric analysis requires addressing common pitfalls:
- Sample selection bias: Ensure that the dataset is representative of the population to avoid biased inferences.
- Multicollinearity: Check correlation matrices to detect highly correlated explanatory variables, and consider dimensionality reduction techniques like PCA.
- Measurement errors: Use validated instruments and robust data sources to minimize errors in variable construction.
By anticipating and mitigating these issues, researchers can improve the reliability and interpretability of their findings.
Limitations of Cross-Sectional Econometrics
Despite its usefulness, cross-sectional econometric analysis has several limitations:
- Lack of temporal dynamics: It cannot detect trends over time.
- Omitted variable bias: Failing to include key factors may skew results.
- Reverse causality: Direction of influence can sometimes be unclear.
Comparing Cross-Sectional and Time-Series Econometrics
While cross-sectional econometric analysis focuses on one time period across multiple subjects, time-series econometrics observes a single subject over several time periods. Each method serves distinct purposes in empirical economics and may be combined in panel data models.
You can explore more about panel data models in our Panel Data Econometrics Guide.
Conclusion
Cross-sectional econometric analysis offers a powerful framework for evaluating economic phenomena using data snapshots. By mastering regression techniques, ensuring correct model specification, and applying best practices, researchers can generate insightful and policy-relevant conclusions.
In the evolving field of econometrics, understanding and leveraging cross-sectional tools remain crucial for academic, governmental, and business applications alike. With increasing availability of high-quality cross-sectional datasets, this methodology continues to play a vital role in evidence-based decision-making and socioeconomic research.