7 Powerful Insights About Generalized Method of Moments Econometrics
By EconometricLinks | May 24, 2025
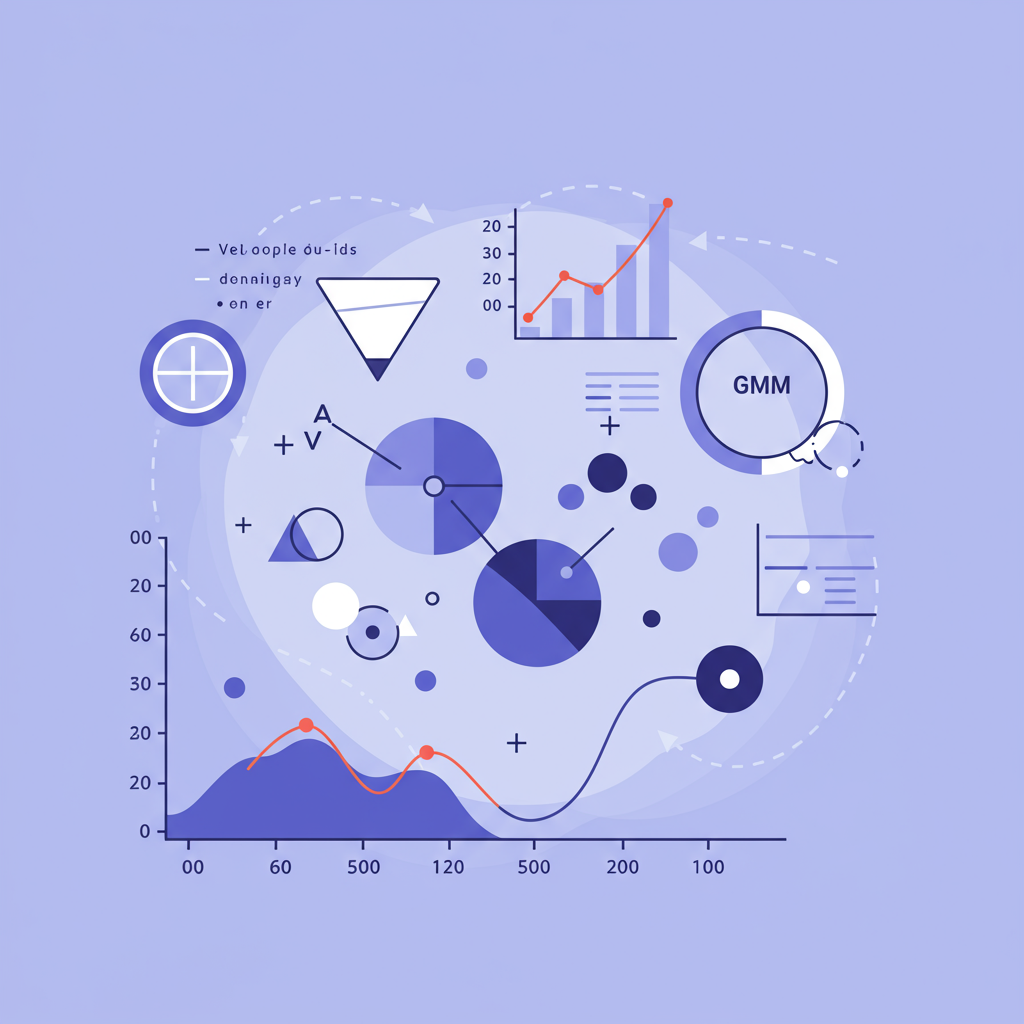
What is Generalized Method of Moments Econometrics?
The generalized method of moments econometrics (GMM) is a flexible and powerful statistical estimation method introduced by Nobel laureate Lars Peter Hansen in 1982. It is widely used in applied econometrics for estimating models when the likelihood function is complex or unknown.
Rather than relying on specific distributional assumptions, GMM leverages moment conditions derived from theoretical economic models. These are expectations (such as means or covariances) that relate observed data to model parameters.
In essence, GMM provides a way to estimate parameters by ensuring that sample moments (calculated from data) align as closely as possible with theoretical expectations derived from economic theory.
How GMM Works in Econometrics
GMM estimation begins by defining moment conditions of the form \( E[g(Z_t, \theta)] = 0 \), where \( Z_t \) are observed data, and \( \theta \) are the parameters to be estimated.
In practical terms, researchers select instruments—variables that are correlated with endogenous regressors but uncorrelated with the error term—to build valid moment conditions. The goal is to find a parameter vector that minimizes the distance between sample and theoretical moments, often using a weighting matrix to control efficiency.
Several statistical software tools support GMM estimation:
For example, in dynamic panel data models, GMM uses lagged variables as instruments to address autocorrelation and endogeneity.
Benefits of Using Generalized Method of Moments Econometrics
Compared to Ordinary Least Squares (OLS) or Maximum Likelihood Estimation (MLE), GMM offers several compelling advantages:
- Robustness to Heteroskedasticity: GMM does not require homoskedastic errors, allowing more flexibility in empirical settings.
- Minimal Distributional Assumptions: It works even when the full distribution of the data is unknown.
- Instrumental Variable Framework: GMM handles endogeneity problems effectively by leveraging instruments.
- Consistency Under Weak Assumptions: It produces consistent estimators as long as moment conditions are valid.
- Applicability to Panel Data: Especially useful in dynamic panels with fixed effects.
Real-World Applications
GMM is a cornerstone in empirical macroeconomics and financial economics. For instance:
- Asset Pricing: In finance, GMM is used to estimate parameters of consumption-based capital asset pricing models (CCAPM).
- Monetary Policy Analysis: Central banks use GMM to model interest rate rules and inflation dynamics.
- Labor Economics: Estimating structural models of wage determination often relies on GMM.
A popular example is the Arellano-Bond estimator, which applies GMM to control for endogeneity in dynamic panel data.
See also: Instrumental Variables in Econometrics.
Common Challenges and Misconceptions
While powerful, GMM is not without pitfalls:
- Instrument Validity: If instruments are weak or invalid, estimates can be severely biased.
- Overfitting with Too Many Instruments: Excessive instruments may distort the Hansen J-test and reduce efficiency.
- Misunderstanding Efficiency: GMM is not always more efficient than MLE unless the optimal weighting matrix is used.
Furthermore, many analysts overlook the need to perform specification tests such as the Sargan or Hansen J-test, which assess the overall validity of the instruments and over-identifying restrictions.
Limitations
GMM’s flexibility comes with trade-offs. Limitations include:
- Sensitivity to Instrument Choice: Poorly chosen instruments can lead to large standard errors or identification problems.
- Complex Implementation: Requires a deep understanding of model theory and econometric tools.
- Finite Sample Bias: Particularly when the number of instruments is large relative to the number of observations.
- No Likelihood-based Inference: GMM lacks a full probabilistic model, making certain statistical tests unavailable.
Conclusion
The generalized method of moments econometrics is a cornerstone of modern empirical research. Its flexibility in handling endogeneity, heteroskedasticity, and complex moment conditions makes it an indispensable tool for economists and data scientists.
However, effective application requires strong theoretical foundations, valid instruments, and careful diagnostic testing. When properly implemented, GMM unlocks deep insights from economic models where traditional methods fall short.
References
- Hansen, L. P. (1982). “Large Sample Properties of GMM Estimators.” Econometrica.
- Wooldridge, J. M. (2010). Econometric Analysis of Cross Section and Panel Data.
- GMM in R